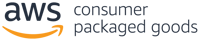
Digital Twins & CPG Manufacturing Transformation
dig·i·tal: of, relating to, or utilizing devices constructed or working by the methods or principles of electronics; [1]also : characterized by electronic and especially computerized technology; : composed of data in the form of especially binary digits
/twin/: something containing or consisting of two matching or corresponding parts.
For the last few years, the term Digital Twin has been at the top of the buzzword list for manufacturers and industrial companies, often meaning different things in different production environments. Most of these organizations have developed Digital Twins to improve operations and product offerings and deliver more business value to their end customers. The concept of digital twins is not new and dates back to the early days of the space program. The Apollo 13 mission in the 1960s is an early use case of using twins to model the state of the damaged spacecraft and bring the astronaut crew safely back to Earth.
In recent years, the core ideas of Digital Twin have been commonly attributed to Michael Grieves of the Digital Twin Institute, who developed the concept throughout the 2000s, and NASA’s John Vickers, who coined the term Digital Twin in 2010. Customers today are seeking to deploy Digital Twins across a broad range of applications, including the design of complex equipment, manufacturing operations, preventive maintenance, precision medicine, digital agriculture, city planning, 3D immersive environments, and most recently, metaverse-type applications.
Digital Twin is often applied broadly to describe any virtual model, including engineering simulations, CAD models, IoT dashboards, or gaming environments. Digital Twins are more than just a marketing term, but rather a new technology that has only become feasible in the past few years with the convergence of at-scale computing, modeling methods, and IoT connectivity.
Let’s first define Digital Twin and how to integrate existing modeling methods into Digital Twins.
What Is a Digital Twin?
A Digital Twin is a living digital representation of an individual physical system that is dynamically updated with data to mimic the true structure, state, and behavior of the physical system, to drive business outcomes.
The four key elements of a Digital Twin are (1) the physical system, (2) the digital representation, (3) the connectivity between the two, and (4) the business outcome. The first element, the physical system itself, can be an individual physical entity, an assembly of physical entities, a physical process, or even a person. It doesn’t necessarily have to be an industrial system but can be a biological, chemical, ecological, or any other system.
The second element is the digital representation, which is the model itself. This is not just a collection of data, such as a data model that represents the structure of the physical system, or an IoT data dashboard that represents the current state of the physical system. A Digital Twin is a model that emulates or simulates the behavior of the physical system, such that when you give it an input, the model returns a response or output. This leads to the third element, connectivity.
A true Digital Twin must be regularly updated with data from the physical system (often using IoT sensors). A validated model provides a snapshot of the behavior of the physical system at a moment in time, and a Digital Twin extends the model to where the physical system’s behavior changes significantly from the original. The frequency of the updates is dictated by the rate at which the underlying changes occur. Some use cases require near real-time updates, whereas others require only weekly updates.
Lastly, the Digital Twin must drive a specific outcome related to some kind of economic or business value. And thus, drive business decisions on the managed process.
The key difference between a Digital Twin and traditional modeling is the information flow between the digital and physical systems. A common misconception of a Digital Twin is that it is simply a more complex, higher fidelity virtual representation. Actually, it is the regular updating that is the key difference and directly impacts both how data is collected throughout the life cycle and how Digital Twins are constructed. A Digital Twin must consume the data streams to understand the present state of the system, learn from and update itself with new observations of the system, and be able to make predictions of the current and future behavior of the system.
For example, a Digital Twin of a carbonated beverage line ingests temperature and pressure data from IoT devices to predict leakage, breaks, quality and non-observable operational elements of the flow. Results of visual inspections from periodic maintenance are used to update the Digital Twin. The Digital Twin is then used to make predictions of downtime and remaining useful life under different operational conditions and maintenance scenarios, enabling the operator to select the best maintenance schedule and plan. Output from the Digital Twin can then be shown to the process owner via a dashboard. Although CAD models, IoT dashboards, 3D renderings, immersive walkthroughs, and gaming environments are not Digital Twins by themselves, they can represent useful visualizations and building blocks of Digital Twin solutions and can be the first steps in a Digital Twin journey.
Why Digital Twins in CPG Now?
Four key technologies are needed to develop and deploy Digital Twins at-scale: data from the physical system, IoT connectivity, modeling methods, and at-scale computing. Each of these technologies have been developed in parallel over the past 20 years. In the 2020s, they have converged to enable Digital Twins at scale in the consumer packaged goods (CPG) industry.
The first technology has to do with measurements of the physical system, which are typically collected using IoT sensors. Performing these measurements have become increasingly affordable thanks to the decrease in the average price of these sensors, which has dropped 50% from 2010 to 2020, and continues to decrease. As a result, measurements that were cost-prohibitive just ten years ago are now becoming a commodity.
The second technology is the ability to transmit this data so it can be analyzed and acted upon efficiently. In recent years, transmission speeds have increased dramatically. If we look at wireless connectivity as a proxy, in 2010, 3G was the de-facto standard at less than 1 Mbps. Throughout the 2010s, it was replaced with 4G at 100 Mbps, and now 5G at 10 Gbps is becoming the norm. That is more than a 10,000x increase in transmission speed. Today, 10 Gbps is considered the milestone threshold for IoT devices as it is fast enough to gather IoT data in near-real time (<10ms latency).
The value of Digital Twins is their ability to use this data to derive actionable insights, which is achieved by modeling the data and leveraging at-scale computing, which are the third and fourth key technologies. The term “model” here is used in multiple contexts. For applications that involve predicting future states and what-if scenario planning, one needs scientific modeling techniques to predict various phenomena and physical behavior such as fluid flow, structural deformation, mix, formulation, weather, and logistics. Methods including machine learning, high performance computing, and hybrid approaches such as physics-inspired neural networks are becoming practical to deploy at scale because of the widespread availability of compute power at the edge in remote production and operational facilities. Another type of modeling can be used for visualization and creating realistic immersive environments. Over the past decade, advancements in spatial computing algorithms that create and manipulate 3D content is enabling immersive augmented reality, virtual reality, and the metaverse.
Lastly, at-scale computing has grown exponentially in recent years. The increase in compute power has occurred both at the chip level and by connecting the chips together to enable massively scalable cloud computing. We’ve reached the point where massive-scale, on-demand compute is becoming a commodity. No longer limited to governments and large corporations, small startups and even individuals can access the necessary compute to innovate, invent new products and services, and improve our daily lives.
What About CPG Use Cases?
There is a wide range of CPG use cases requiring different services, technologies, and data needed to enable them. Let’s consider a leveling index as follows: (1) Descriptive, (2) Informative, (3) Predictive, and (4) Living.
L1 Descriptive focuses on the engineering design and the visual representation of the physical system and its structure. It can be a 2D engineering diagram (such as a process or P&ID diagram), a building information model (BIM), or a complex high-fidelity 3D/AR/VR model. It also includes engineering analysis performed such as 1D analysis, systems dynamics, computational fluid dynamics, and structural mechanics. The purpose is to understand the design of the physical system and its components.
L2 Informative focuses on integration of IoT sensor and maintenance data from the physical system and displaying it to the end-user in a context-relevant manner, such as a 2D dashboard or a 3D contextual visualization (e.g., its state). It enables the end user to understand the present condition of the physical system and can include simple analytics to trigger alarms. In the CPG world, this is the domain of IoT and asset management integrated with enterprise asset management (EAM) or enterprise resource planning (ERP) systems to show asset configuration, maintenance history, and upcoming work orders on a single pane of glass.
L3 Predictive focuses on predictions of unmeasured quantities (e.g., virtual sensors, machine learning based anomaly detection), as well as using predictive models to understand future states under continued operations where the future behavior is the same as past behavior. These models can either be based on scientific first principles, purely data-driven (e.g., using AI/ML), or a hybrid of the two.
L4 Living focuses on updatable models to drive actionable insights at the individual entity level that can be aggregated to the fleet level if desired. The key distinction between L3 Predictive and L4 Living is the ability for the model itself to be updated based on the data from the physical entity and environment. From a business perspective, the model update capability of an L4 Digital Twin extends its use to timescales over which the behavior of the physical system changes significantly, whereas an L3 Digital Twin is useful for predictions at a moment in time (or very short time-scales thereafter). One way to understand this is that a predictive model trained on historical data is, by definition, inaccurate the day it is deployed, since the change in the physical system is not in the training data set. Using this inaccurate model for a forward prediction, in practice, results in a large propagation of error such that the prediction becomes useless over time.
Digital Twin at Coca-Cola Icecek
Coca-Cola Icecek (CCI) is the 6th-largest bottler of Coca-Cola products by sales volume in the world. To clean production equipment, the company uses a “clean-in-place” (CIP) process with the goal of reducing water and energy usage by 12%. However, optimizing the CIP process is currently more art than science and presents the following challenges including, (1) Lack of visibility to changes in CIP performance, (2) Difficult to gather insights from millions of data points, and (3) Variability in measurement due to process or human error.
Using AWS IoT and machine learning services, CCI implemented a Digital Twin solution (“Twin Sense”) in two months to provide visibility of leading indicators to CIP performance, enable operators and quality team to take actions on process failures, identify the root cause of problems and garner insights, and standardize, benchmark, and optimize CIP processes.
In six weeks, CCI was able to impact energy use and water consumption. CCI reduced the environmental impact of CIP process and saved 1,236 kW of energy, saved 560 m3 of water, saved 2,400 L of cleaning agent, optimized CIP process time and cost performance, and improved CIP process visibility for plant operators.
This concept was validated and a commercial model for scale at 30 other facilities was developed.
What’s Next!?
Many CPG brands are still early in their Digital Twin journey. They are working hard to connect their data across disparate sources and be able to contextually visualize that data in a dashboard or an immersive environment. The first applications have been highly customized and only make financial sense for high-value use cases. Over the next few years, expect to see services lower their costs and simplify deployment. This commoditization will drive adoption across a broad range of everyday contextual visualization use cases.
In parallel, expect to see advanced predictive modeling methods become more readily accessible for high-value use cases. Today, these methods are currently available and used by niche R&D and advanced analytics teams. Eventually, these methods will also become mainstream and easily applied for everyday use cases, allowing anyone to make an L4 living Digital Twin.