Unilever Targets Growth in Emerging Markets through Analytics
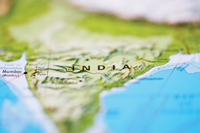
Despite a steady growth of large modern retailers, traditional trade still represents a significant share of retail sales in emerging markets. The key to growth is to empower the field force with intelligent market information. The challenge, however, is to do so in the absence of point-of-sale (POS) data to help identify consumer-buying behaviors and patterns. Additionally, generating specific information for each unique outlet becomes important. This can be a herculean task, considering the magnanimity of the data.
In response, Hindustan Unilever (www.hul.co.in) launched a strategic analytics initiative called “Project iQ”, which aims to create “perfect stores” by improving on-shelf availability, reducing stocks-outs and enhancing assortment. As part of Project iQ, and with Mindtree Limited (www.mindtree.com) as a solution partner, Unilever Hindustan used measurable science to generate specific outlet product-level decisions that can be driven in the field. The solution was delivered through a dedicated analytics cell called “Decision Support Group”, and it has applicability across channels, geographies and outlets of varied sizes. Neighborhood behavior is also used as a key influencer in generating recommendations. Solution capabilities include:
- Cross-Sell Recommendations: Customized predictive models are built using outlet-level historic transactional data. The outcome is a score indicating the selling propensity of a product that is currently not selling. Decisions made on the field can result in assortment and revenue increases.
- Stock-Out Analysis: The prediction of stock-outs is done based on the estimation of key parameters, like closing stock and selling rate, to predict the stock availability on a given day. Done with reasonable accuracy and undertaking proactive replenishment can lead to significant growth in on-shelf availability.
FAST FACTS
Words of Wisdom
“No human being can look at data from a million and a half outlets and come up with the answers; the designed logic is to build an algorithm and come up with certain recommendations of the system.” — Nitin Paranjpe, CEO and managing director, Hindustan Unilever
Data Differences
Emerging markets are characterized by a dependence on traditional trade, which does not capture tertiary or POS data. Instead, data sources include secondary sales data; the product, distributor and store masters; and field audit data for stock-out analysis.
The Perfect Store
Hindustan Unilever’s “Perfect Stores” program focuses on better availability and visibility of key brands in retail stores. The program is supported by Project iQ, which has evolved to become a global analytics initiative.
Analytics Pay Off
A tool created by Project iQ’s solution partner Mindtree Limited led to new cross-sell recommendation capabilities that resulted in increased assortment, thereby generating significant incremental revenue for Hindustan Unilever.